Autonomous high-accuracy analysis of prostate cancer biopsies
A framework for automated detection of prostate cancer on histopathology slides.
Technology No. 2019-015
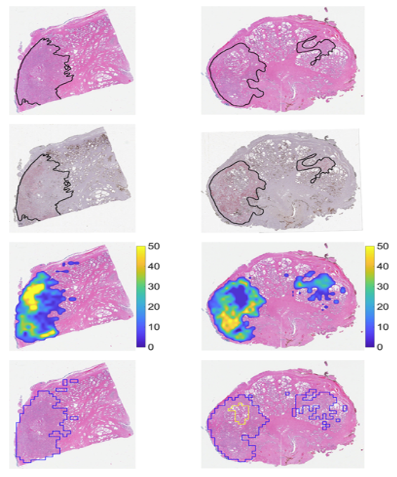
1
IP Status: US Patent Issued / U.S. Patent No. 11,631,171
Working prototype available.
Applications
- Computer-aided cancer detection
- Software as a service (SaaS) for detection, grading of cancer
- Cancer pathology
- Model to predict cancer using non-invasive tools such as MRI
Key Benefits
- Saves significant time and cost
- High accuracy of detection and grading, comparable to that of trained pathologists
- Eliminates user error and subjectivity
Autonomous Annotation of Histologically-Stained Samples
This technology is an automated, machine learning-based tissue analysis software that can predict the percentage of cancerous epithelium on histologically-stained samples, and reliably detect prostate cancer. This technology significantly reduces time and cost associated with manual annotation (i.e., detection and delineation) of tissue sample pathology slides often done by pathologists. The regression model developed here accurately predicts the percentage of cancerous epithelium within a sample, and a threshold is applied to reliably obtain a binary label of cancer vs. non-cancer sample.
Algorithm Derived from High-Confidence Ground Truth
The gold standard for obtaining the ground truth to train a predictive model requires the manual annotation of ex vivo prostatectomy samples. Such manual annotations performed by trained pathologists are very time-consuming and prone to error due to subjectivity. The method described here automates the laborious process of cancer annotation, saving significant time while achieving cancer detection accuracy comparable to that of a trained pathologist. This automated cancer annotation algorithm uses colorimetric information obtained from more than one type of staining (including morphology, architecture, and pathologic state of tissue), thereby increasing its predictive accuracy. The ground truth used to train the predictive model is derived from manual annotation of samples at high resolution, which ensures high confidence in its accuracy.Phase of Development
TRL: 4-5Working prototype available.
Desired Partnerships
This technology is now available for:- License
- Sponsored research
- Co-development
Please contact our office to share your business’ needs and learn more.
Researchers
- Gregory J Metzger, PhD Professor, Department of Radiology
- Joseph Koopmeiners, PhD Professor, Division of Biostatistics
-
swap_vertical_circlelibrary_booksReferences (1)
- Ethan Leng, Jonathan C. Henriksen, Anthony E. Rizzardi, Jin Jin, Jung Who Nam, Benjamin M. Brassuer, Andrew D. Johnson, Nicholas P. Reder, Joseph S. Koopmeiners, Stephen C. Schmechel & Gregory J. Metzger (2019), Signature maps for automatic identification of prostate cancer from colorimetric analysis of H&E- and IHC-stained histopathological specimens, Sci Rep, 9, 6992 (2019)
-
swap_vertical_circlecloud_downloadSupporting documents (1)Product brochureAutonomous high-accuracy analysis of prostate cancer biopsies.pdf