Assisted machine learning architecture
A disruptive machine learning architecture invented for privacy-sensitive entities to collaborate with each other without sacrificing the quality of gained intelligence.
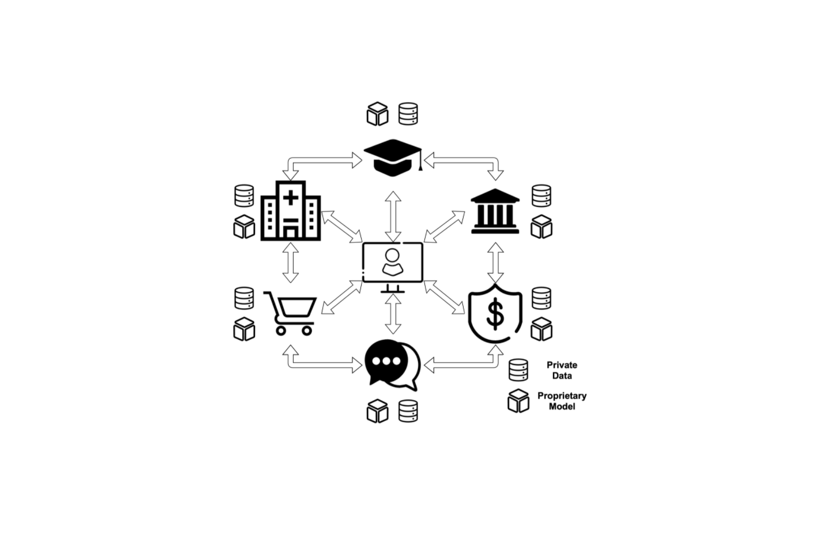
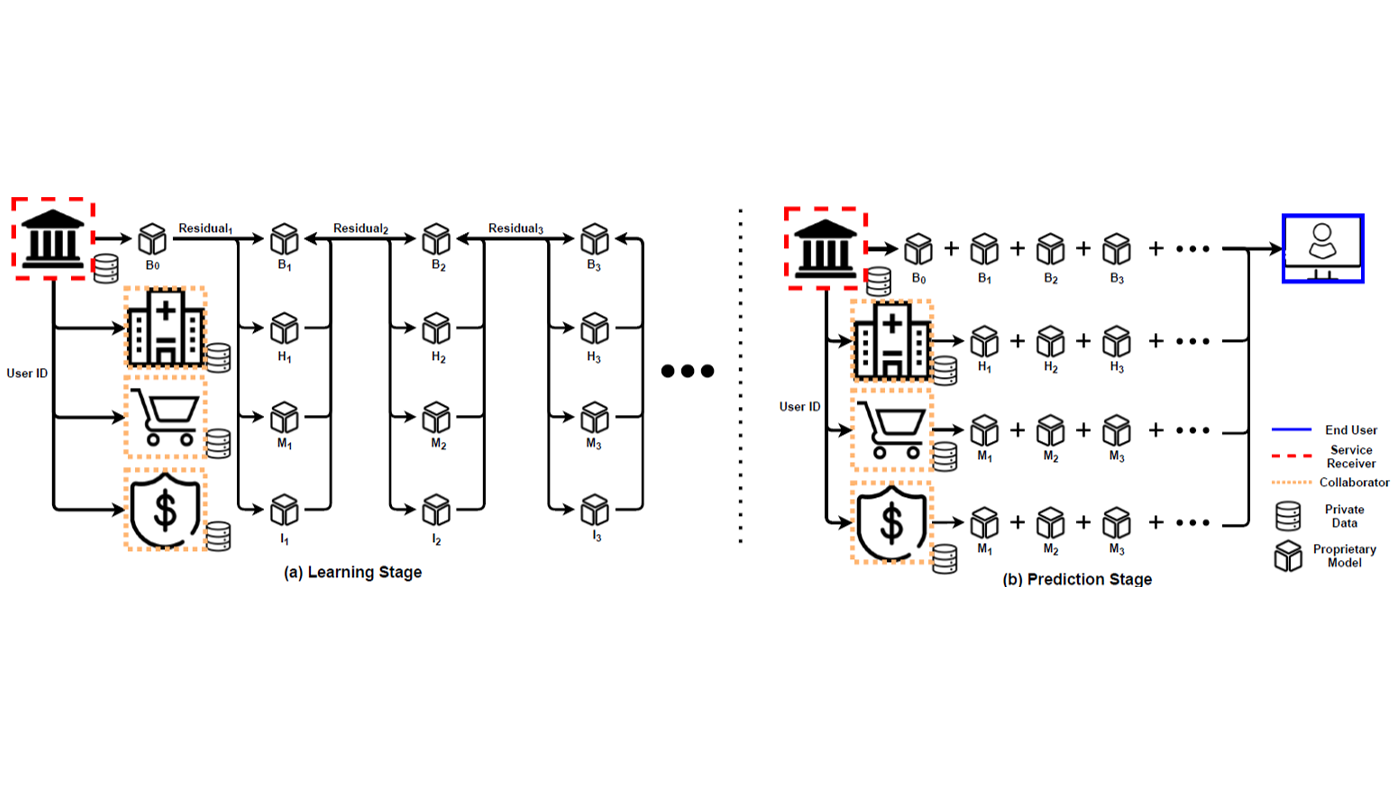
US Patent Pending; Application #: 17/248,845
Provisional Patent Application Filed; Application #: 63/202,385
Applications
- Machine Learning
Machine learning architecture with high data and model privacy
Concerns of data security and privacy have led to more stringent regulations on the use of data in machine learning. Successful conventional machine learning architectures provide intelligence from user data sets but often require disclosure of that data. Collaborating with privacy is one of the most significant challenges in
contemporary machine learning. There, designing machine learning architectures that facilitate not only accuracy, but also privacy and data security is of high interest. In addition, there is also a growing demand for protecting the learner units that manage data.
To address these issues, researchers at the University of Minnesota have developed Assisted Machine Learning Architecture, a disruptive technology invented for privacy-sensitive entities to collaborate without sacrificing the quality of gained intelligence. In Assisted Machine Learning Architecture, an entity/learner assists another entity's machine learning by iteratively exchanging non-private statistics such as confidence scores. This newly developed methodology can be applied to a broad range of machine learning types such as regression and classification, and can allow assistance among numerous entities in a computationally efficient manner.
This invention simultaneously addresses two challenges in the current machine learning ecosystem. 1) Privacy: the state-of-the-art privacy focuses only on data. This technology offers several methods and software architecture that focus on the protection of each learner unit, models as well as data. 2) Design of decentralized machine learning architecture: Each learner has the flexibility of assisting or being assisted by others, which simultaneously facilitates flexibility, fairness, privacy, and personalization, a design drastically different from previous state-of-the-art architectures (such as the current popular Federated learning, a centralized learning architecture, advocated by multiple influential technology companies).
Assisted Machine Learning Architecture is applicable in privacy-aware, transmission-economical, and decentralized learning scenarios such as collaborations between clinics or hospitals, services among financial institutions, distributed learning among smart devices/mobile apps, and between IT companies. Additional applications include machine assisted learning among cameras that observe the same objects, among clinical divisions that record the same patients, etc.
Key Benefits & Differentiators
-
Data:
- Data are not exchanged or released
- Model architectures and parameters are not exchanged
- Only task-relevant statistics are exchanged Models:
- Autonomously-chosen models without a global model
- Personalized learning goals Architecture:
- Any individual module can be either service provider or user
- Adversarial modules allowed From the user's perspective:
- Module-level privacy
- Private model & task objective, as well as private data
- Active querying of useful information to assist personalized learning From the service provider's perspective:
- Module-level privacy
- Private model as well as private data
- Active responding to benign queries to assist each user's personalized learning in a black-box manner
Phase of Development
TRL: 3-4Software prototype has been developed.
Desired Partnerships
This technology is now available for:- License
- Sponsored research
- Co-development
Please contact our office to share your business’ needs and learn more.
Researchers
- Jie Ding, PhD, Assistant Professor, Statistics
-
swap_vertical_circlelibrary_booksReferences (3)
- Xian, Xun, Xinran Wang, Jie Ding, and Reza Ghanadan. , "Assisted learning: A framework for multi-organization learning.", arXiv preprint arXiv:2004.00566 (2020).
- Wang, Xinran, Yu Xiang, Jun Gao, and Jie Ding. , "Information laundering for model privacy.", arXiv preprint arXiv:2009.06112 (2020).
- Diao, Enmao, Jie Ding, and Vahid Tarokh. , "Gradient Assisted Learning.", arXiv preprint arXiv:2106.01425 (2021).
-
swap_vertical_circlecloud_downloadSupporting documents (1)Product brochureAssisted machine learning architecture.pdf